Alberto Acerbi https://orcid.org/0000-0001-5827-8003 alberto.acerbi@unitn.it and Joseph M. Stubbersfield https://orcid.org/0000-0001-8966-4679
Edited by Marcus Feldman, Stanford University, Stanford, CA; received August 10, 2023; accepted September 26, 2023
October 26, 2023
120 (44) e2313790120
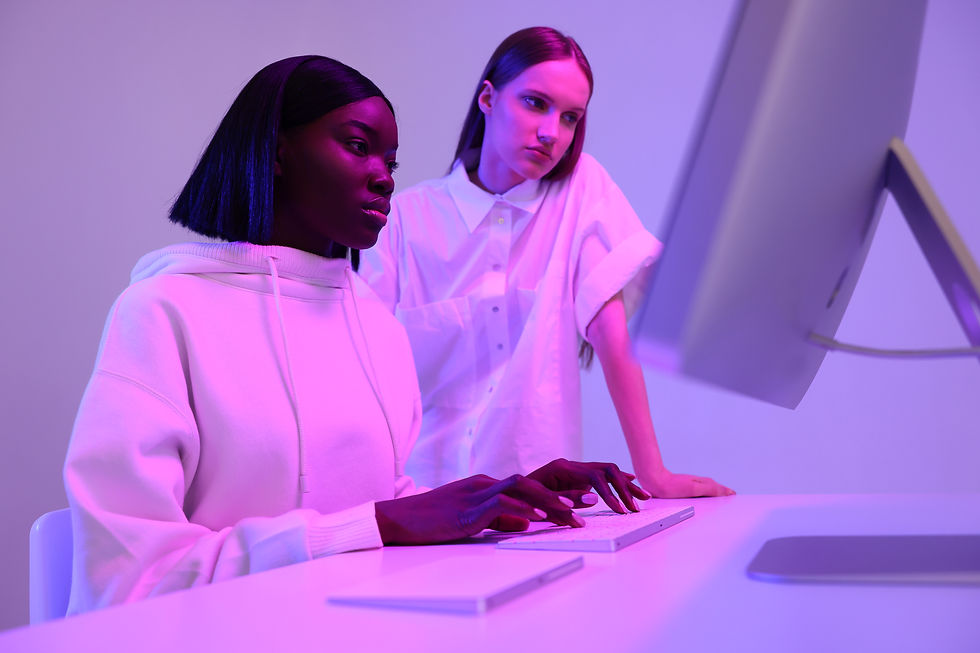
Use of AI in the production of text through Large Language Models (LLMs) is widespread and growing, with potential applications in journalism, copywriting, academia, and other writing tasks. As such, it is important to understand whether text produced or summarized by LLMs exhibits biases. The studies presented here demonstrate that the LLM ChatGPT-3 reflects human biases for certain types of content in its production. The presence of these biases in LLM output has implications for its common use, as it may magnify human tendencies for content which appeals to these biases.
Abstract
As the use of large language models (LLMs) grows, it is important to examine whether they exhibit biases in their output. Research in cultural evolution, using transmission chain experiments, demonstrates that humans have biases to attend to, remember, and transmit some types of content over others. Here, in five preregistered experiments using material from previous studies with human participants, we use the same, transmission chain-like methodology, and find that the LLM ChatGPT-3 shows biases analogous to humans for content that is gender-stereotype-consistent, social, negative, threat-related, and biologically counterintuitive, over other content. The presence of these biases in LLM output suggests that such content is widespread in its training data and could have consequential downstream effects, by magnifying preexisting human tendencies for cognitively appealing and not necessarily informative, or valuable, content.
Comments