Anton Korinek, JOURNAL OF ECONOMIC LITERATURE
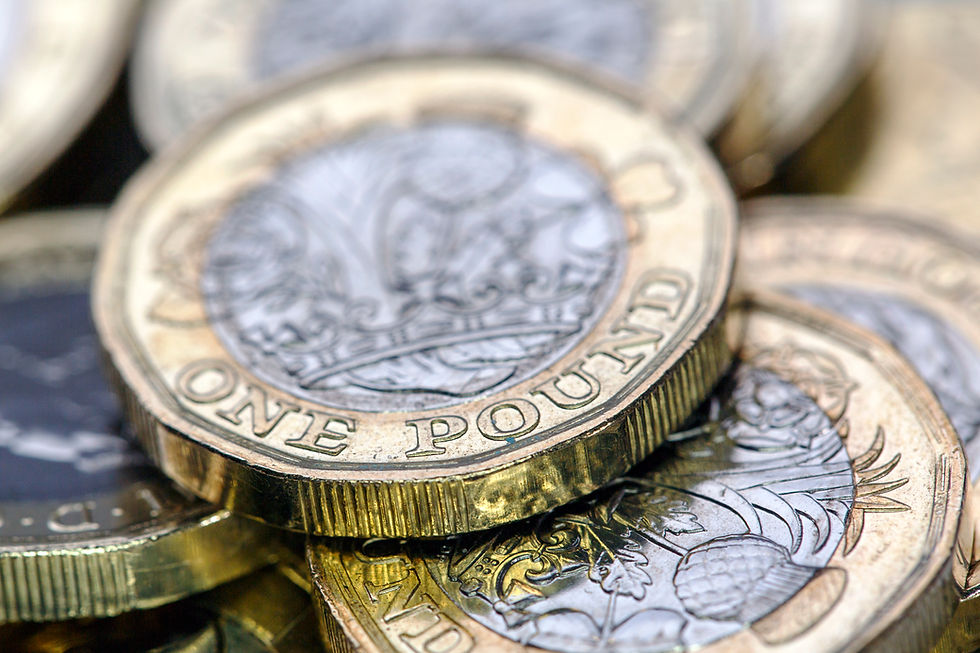
The landscape of generative artificial intelligence (AI) has seen rapid evolution, significantly impacting economic research. Since the original publication of this article in the *Journal of Economic Literature* (Korinek, 2023), advancements in large language models (LLMs) have introduced various innovations and new use cases. This report summarizes the main innovations and updated use cases of cutting-edge LLMs in economic research, focusing on six domains: ideation and feedback, writing, background research, coding, data analysis, and mathematical derivations.
Recent progress in LLMs is marked by improved performance, expanded context windows, enhanced recall, faster processing, and reduced costs. Significant updates from the leading AI labs have incorporated vision capabilities and real-time sound processing. As of May 2024, OpenAI's GPT-4o stands as the most capable publicly available LLM, featuring a GPT store within the ChatGPT app. Anthropic's Claude 3, released in March 2024, excels in writing capabilities, while Google DeepMind's Gemini Pro 1.5, released in January 2024, offers the largest context window, capable of processing up to 1 million tokens.
The leading LLMs from OpenAI, Anthropic, and Google DeepMind exhibit similar performance levels, with OpenAI holding a slight lead. Proprietary models like GPT-4o, Claude 3, and Gemini Pro 1.5 integrate language processing with visual information processing, enabling comprehensive responses based on both text and images. These models are accessible via web-based chatbots, real-time voice assistants, web-based experimentation platforms, and APIs. Additionally, smaller, open-source models have been released, providing users with options for privacy-sensitive applications.
Use Cases in Economic Research
1. Ideation and Feedback:
- LLMs assist in generating and evaluating research ideas, providing a form of creativity that complements human researchers. They are particularly useful for brainstorming and evaluating ideas, highlighting the pros and cons of different hypotheses.
2. Writing:
- LLMs can synthesize text from bullet points, convert equations to LaTeX, edit for grammatical and stylistic improvements, and generate titles, headlines, and social media posts. These capabilities streamline the writing process and enhance productivity.
3. Background Research:
- LLMs excel at summarizing large bodies of text, identifying relevant literature, explaining new concepts, and translating texts. Their ability to process entire papers and videos extends their utility in comprehensive background research.
4. Coding:
- LLMs can assist with coding tasks by generating code snippets, debugging, and providing explanations for complex code segments. This functionality is particularly beneficial for researchers with limited programming expertise.
5. Data Analysis:
- Advanced data analysis capabilities in LLMs allow for the execution of Python code within the ChatGPT interface, facilitating tasks such as file conversions, regressions, and simulations.
6. Mathematical Derivations:
- LLMs can perform mathematical derivations, helping researchers with complex calculations and model simulations.
While LLMs offer numerous benefits, they also present challenges, such as data confidentiality, model biases, and the potential for over-reliance on automation. Ensuring the ethical use of LLMs and addressing these challenges is crucial for maximizing their positive impact on economic research. The integration of generative AI and LLMs in economic research is poised to significantly enhance productivity and innovation. As these technologies continue to evolve, their capabilities will further expand, offering new opportunities and challenges for researchers. Adopting these tools thoughtfully and ethically will be key to leveraging their full potential in the economic research domain.
Comentários